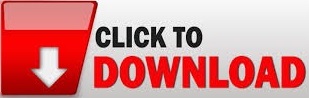
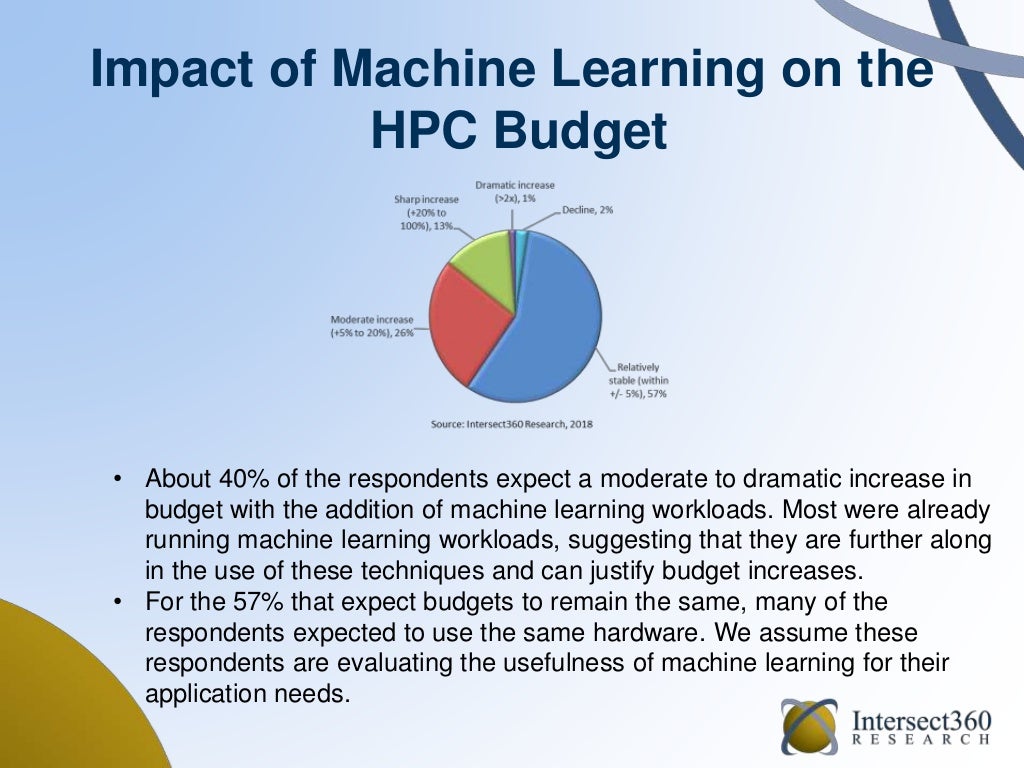
In this work, we propose HPCFAIR, a modular, extensible framework to enable AI models to be Findable, Accessible, Interoperable, and Reproducible (FAIR). The primary challenge is a lack of an ecosystem to reuse and reproduce the models and datasets. Given multiple efforts in this arena, there are often duplicated efforts when existing rich data sets and ML models could be leveraged instead. A significant interest in the scientific community also involves leveraging machine learning (ML) to run high-performance computing applications at scale effectively.
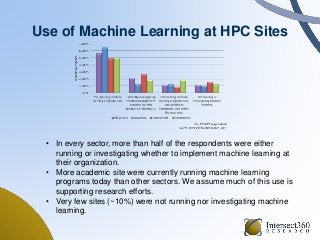
HPCFAIR: Enabling FAIR AI for HPC ApplicationsĪrtificial Intelligence (AI) is being adopted in different domains at an unprecedented scale. Finally, we show that we can reduce by an order of magnitude the number of evaluations necessary to find the most optimal region in the hyperparameter space and reduce by two orders of magnitude the throughput for such HPO process to complete.
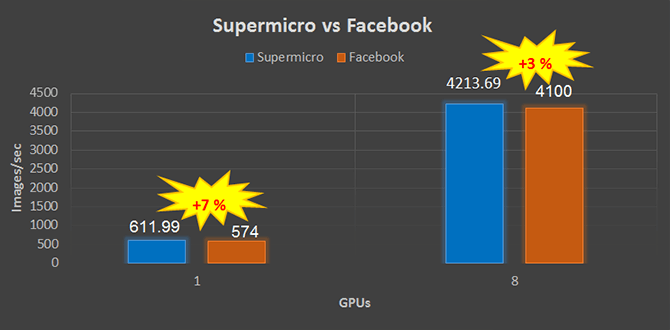
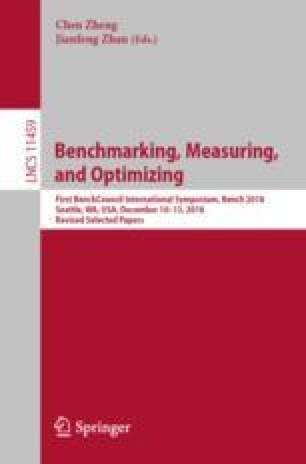
#Hpc ai benchmark software#
We demonstrate various software features on time-series prediction and image classification problems as well as a scientific application in computed tomography image reconstruction. HYPPO is implemented in Python and can be used with both TensorFlow and PyTorch libraries. Using asynchronous nested parallelism, we are able to significantly alleviate the computational burden of training complex architectures and quantifying the uncertainty. Unlike other hyperparameter optimization methods, HYPPO uses adaptive surrogate models and directly accounts for uncertainty in model predictions to find accurate and reliable models that make robust predictions. We present a new software, HYPPO, that enables the automatic tuning of hyperparameters of various deep learning models. Vincent Dumont (Lawrence Berkeley National Laboratory) HYPPO: A Surrogate-Based Multi-Level Parallelism Tool for Hyperparameter Optimization Furthermore, we characterize each benchmark with compute, memory and I/O behaviours to parameterize extended roofline performance models. We present the results from the first submission round, including a diverse set of some of the world's largest HPC systems, along with a systematic framework for their joint analysis and insights on implementations. In this paper, we introduce MLPerf HPC, a benchmark suite of large-scale scientific machine learning training applications driven by the MLCommons(TM) Association. MLPerf(TM) is a community-driven standard to benchmark machine learning workloads, focusing on end-to-end performance metrics. There is a critical need to understand fair and effective benchmarking of machine learning applications that are representative of real-world scientific use cases. High performance computing systems are pushing the frontiers of performance with a rich diversity of hardware resources and massive scale-out capabilities. Scientific communities are increasingly adopting machine learning and deep learning models in their applications to accelerate scientific insights. Steven Farrell (Lawrence Berkeley National Laboratory) and others MLPerf HPC: A Holistic Benchmark Suite for Scientific Machine Learning on HPC Systems
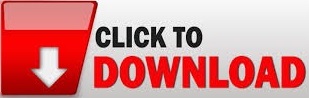